
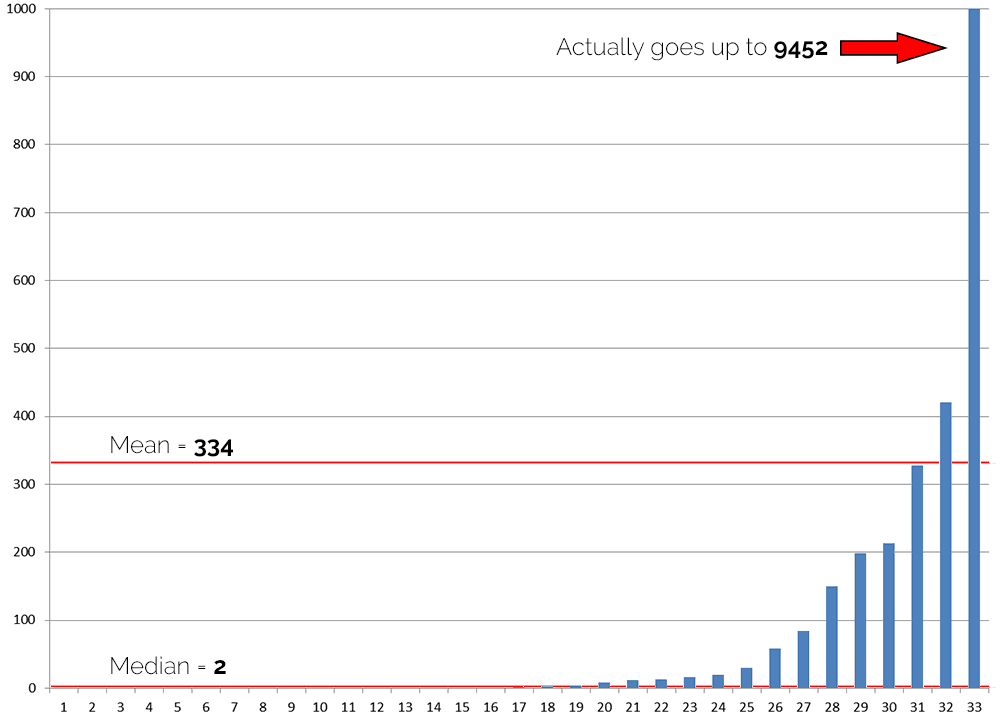
The development of innovations in all the research and development (R&D) fields is leading to a huge increase of patent data. We also find the crossover advantage, i.e., interconnection between two chain networks can make the related individuals possessing higher social influences, i.e., their messages can be forwarded by relatively more others.We obtained these results theoretically and validated them by simulations, which help better understand the reciprocity between social networks and information diffusion. Moreover, we observe the emergence of social stratification based on the new model, i.e., the true messages initially posted by an individual closer to the smart one can be forwarded by more others, which is enhanced by the self-learning mechanism.

Based on such a simple self-learning mechanism, we prove that a social network can indeed become smarter, in terms of better distinguishing the true message from the false one.
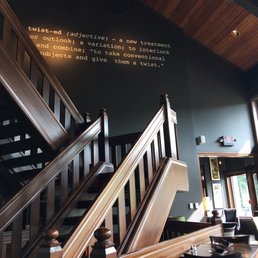
Since social networks consist of human individuals, who have self-learning ability, in such a way that the trust of an individual to one of its neighbors increases (or decreases) if this individual received a true (or false) message from that neighbor. Specifically, we first introduce a novel information diffusion model on social networks, by considering two types of individuals, i.e., smart and normal individuals, and two kinds of messages, true and false messages. In this paper, we propose a new framework for understanding this reversing effect. However, the opposite question, i.e., how does information diffusion process rebuild social networks, has been largely ignored. It is widely believed that social networks play important role in the process of information diffusion. In this big data era, more and more social activities are digitized thereby becoming traceable, and thus the studies of social networks attract increasing attention from academia. The chapter is an effort in the direction of online social network analysis that explores the basic methods that can be process and analyze the network with a suitable approach to yield knowledge. Local features include properties of nodes like degree of the node (in-degree, out-degree) while global feature process the path between nodes in the entire network. This is referred to as social network analysis, and the features exploited usually are local and global both plays significant role in processing and computation. Processing of online social networks structural features yields fair knowledge which can be used in many of recommendation and prediction systems. The structure of social networks is comprised of nodes and edges whereas users are entities represented by node and relationships designated by edges. Millions of users are appending to it and enriching the nature and behavior, and the information generated has various dimensional properties providing new opportunities and perspective for computation of network properties. Finally, we present two use cases that can highly benefit from the knowledge on k-bridges detected through our approach.Įxpansion of online social networks is rapid and furious. We also propose two further specializations of k-bridges, regarding Reddit and the network of patent inventors, to prove that the knowledge on k-bridges we initially found in Yelp is not limited to this social network. In addition, we investigate the relationship between k-bridges and the main centrality measures in the macro-categories of Yelp. We also analyze the relationship between k-bridges and power users. Then, we verify if there exists an influence exerted by k-bridges on their friends and/or on their co-reviewers. In particular, we investigate how some basic characteristics of Yelp k-bridges vary against k (i.e., against the number of macro-categories which the businesses reviewed by them belong to). Then, we analyze the specialization of this concept and algorithm in Yelp and we extract several knowledge patterns about Yelp k-bridges. In this paper, we introduce the concept of k-bridge (i.e., a user who connects k sub-networks of the same network or k networks of a multi-network scenario) and propose an algorithm for extracting k-bridges from a social network.
